In this article, I comrehensively explain the differences between Understanding Full Mediation and Partial Mediation
There has been a great deal of confusion regarding statistical terms like mediation, moderation, interaction, confounding, synergy effect, intervening variable, control variable, co-variate etc.
In this series of articles, I’ll briefly describe each of these term by providing suitable examples. I’ll try to simplify the difference and similarities between various terms. Techniques for identification, consequences of these effect and techniques to deal with these effects are different [we may discuss it later].
In the first article, we will understand the concept of Full/Partial Mediation Effect.
1. Main/Direct Effect:
The predictor variable X predicts the response variable Y. This is the case of simple regression. We can also refer the impact of predictor X on Y as main/direct effect. Although, this terminology is not very useful here.
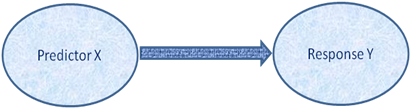
Figure_1 Simple regression
2. Mediation Effect:
The predictor variable X predicts the mediator M (a third intervening variable, also known asmediating variable), which in turn predicts the response Y. In other words, a mediation effect occurs when the third variable (mediator, M) carries the influence of a given predictor variable X to a given response variable Y.
The mediating variable can be labeled as Intervening or intermediary ormediating or surrogate Variable but normally, we use this term when we have Complete Mediating Effect.
Mediation effect can be broadly classified as full mediation and partial mediation. Let’s understand the difference between the two:
(a) If predictor variable X in does not have a direct significant impact on response variable Y but it has a significant impact on moderator M, which also has a significant impact on response variable Y, this is known as a case of complete/full mediation. The relationship between predictor, moderator and response variable can be represented by the following figure.

Figure_2 (a) Full Mediation
(b) If predictor variable X in has a direct significant impact on response variable Y and it also has a significant impact on moderator M, which has a significant impact on response variable Y, this is known as a case of partial mediation. The direct impact of predictor X on response variable Y is known as direct effect, whileThe impact of predictor X on response variable Y through the third variable ‘mediator’ is known as indirect effect. Adding a mediator makes direct effect get smaller, as some of the impact (of predictor on response) is being captured by a third variable ‘mediating variable’.
The indirect effect can also be known as Intervening or intermediary ormediating or surrogate effect.
The relationship between predictor, moderator and response variable can be represented by the following figure
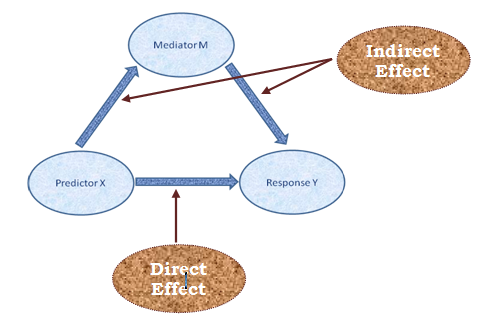
Figure_2 (b) Partial Mediation
Let’s understand both concepts by using some examples:
Example_1:
Let’s take a research issue – does the market orientation in organization have any impact on organizational performance? It means that the researcher is going to test the following model:
Model_1: Market Orientation in organization à Organizational performance ?

Figure_3 (a) Model_1: Simple Regression
Assume that such relationship exists and it is coming to be significant. It is simple regression model.
Later on, it is found that Market Orientation in organization impacts employees’ attitude and that in return has impact on Organizational Performance. Therefore, the researcher develops the following model:
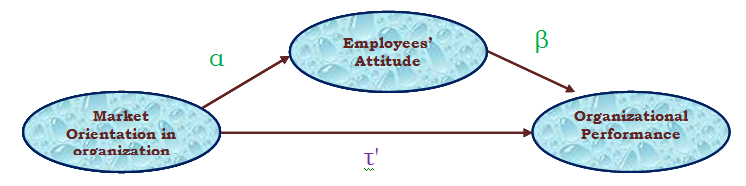
Figure_3 (b) Model_2: Partial Mediation
Researcher can conclude the presence of partial mediation effect (with employees’ attitude as an mediating variable), if all three relationships (denoted by α, β and τ’) are statistically significant. [1]Statistical techniques of testing mediation effects will be discussed later.
Now, suppose the researcher tested the above model and found that Market Orientation in organization has a significant impact on Employees’ Attitude and in turn, Employees’ Attitude has a significant impact on Organizational Performance but Market Orientation in organization does not have a direct significant impact on Organizational Performance. In that case the model reduced to the following:
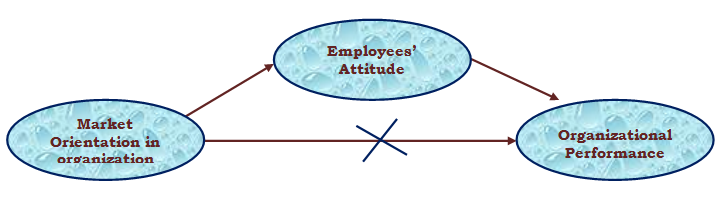
Or we may represent the model as

It means that Market Orientation in organization impacts (statistically significant) Employees’ Attitude, which again impacts (significantly) Organizational Performance and it is called Complete Mediation.
Example_2: Complete mediation
Predictor X: time spent outdoors, mediator M: being bitten by mosquitoes and Response Y: contracting Zika virus.
This assumes that Zika is only delivered through mosquitoes. One can see that time spent outdoors might increase your chance of contracting Zika, but only through being bitten.
Example_3: Complete mediation
Predictor X: Mother’s time spent with abuser, mediator M: depression, Response Y: child behavior problems
This assumes that child behavior problems are present only due to depression and that depression is associated with only mother’s time spent with abuser.
Example_4: Partial mediation
Predictor X: Positive life event, mediator M: Life style and Response Y: subjective happiness
This assumes that positive life event causes subjective happiness, but the impact is mediated by a third variable ‘life style of the person’.
Example_5: Partial mediation
Predictor X: Age, mediator M: Weight and Response Y: Blood Pressure
This assumes that blood pressure increase with increase in age of the patient but his/her age also mediates the effect. As age & weight are also positively associated and increased weight also causes increase in blood pressure.
Note Points:
- In all these examples, the effect of other variables is ignored.
- These examples are provided, so that reader can grasp the concept of full and partial mediation simply.
- Same variable can act as a predictor or mediator or response variable in different contexts. Researcher should establish/test any model which is supported by existing literature.