Levels of measurement, also called scales of measurement, tell you how precisely variables are recorded. In scientific research, a variable is anything that can take on different values across your data set (e.g., height or test scores).
There are 4 levels of measurement:
- Nominal: the data can only be categorized
- Ordinal: the data can be categorized and ranked
- Interval: the data can be categorized, ranked, and evenly spaced
- Ratio: the data can be categorized, ranked, evenly spaced, and has a natural zero.
Depending on the level of measurement of the variable, what you can do to analyze your data may be limited. There is a hierarchy in the complexity and precision of the level of measurement, from low (nominal) to high (ratio).
Nominal, ordinal, interval, and ratio data
Going from lowest to highest, the 4 levels of measurement are cumulative. This means that they each take on the properties of lower levels and add new properties.
Nominal level | Examples of nominal scales |
---|---|
You can categorize your data by labelling them in mutually exclusive groups, but there is no order between the categories. |
|
Ordinal level | Examples of ordinal scales |
---|---|
You can categorize and rank your data in an order, but you cannot say anything about the intervals between the rankings. Although you can rank the top 5 Olympic medallists, this scale does not tell you how close or far apart they are in number of wins. |
|
Interval level | Examples of interval scales |
---|---|
You can categorize, rank, and infer equal intervals between neighboring data points, but there is no true zero point. The difference between any two adjacent temperatures is the same: one degree. But zero degrees is defined differently depending on the scale – it doesn’t mean an absolute absence of temperature. The same is true for test scores and personality inventories. A zero on a test is arbitrary; it does not mean that the test-taker has an absolute lack of the trait being measured. |
|
Ratio level | Examples of ratio scales |
---|---|
You can categorize, rank, and infer equal intervals between neighboring data points, and there is a true zero point. A true zero means there is an absence of the variable of interest. In ratio scales, zero does mean an absolute lack of the variable. For example, in the Kelvin temperature scale, there are no negative degrees of temperature – zero means an absolute lack of thermal energy. |
|
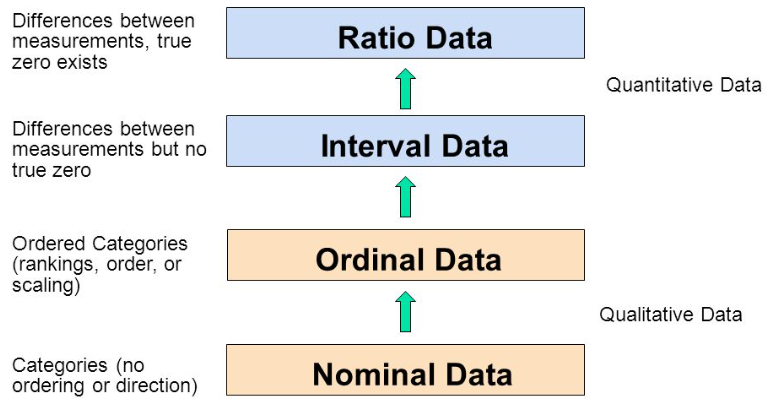
Consult a Data Analyst
We are a team of dedicated data analysts and econometricians that have competent experience in data modelling, statistical tests, hypothesis testing, predictive analysis and interpretation
- admin@datapott.com